Laslo Dinges
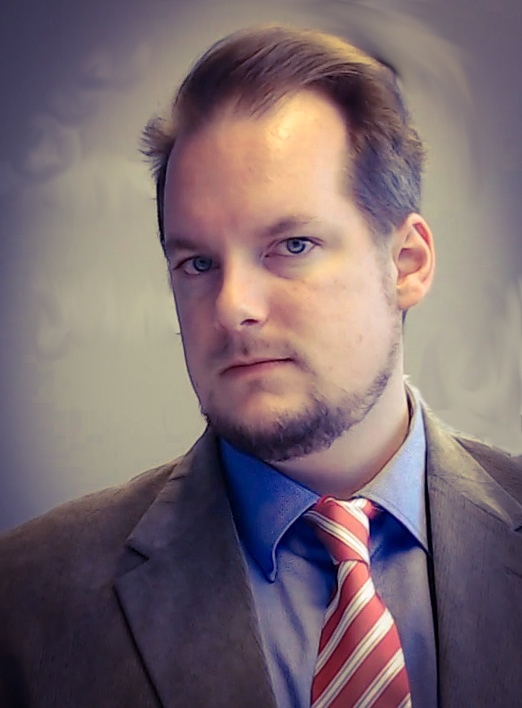
Dr.-Ing. Laslo Dinges
Postdoc
Research Focus
- Pattern Recognition
- Segmentation based and holistic handwriting recognition
- Statistical model based synthetesis of handwriting samples
- Document analysis
- Optical 3D Surface Inspection
- Realtime analysis of facial expressions (in the context of human-robot interaction)
Projects
Supervision of Bachelor/Master Theses
- "Fast and Precise Binary Instance Segmentation of 2D Objects for Automotive Applications", Master Thesis at OvGU Magdeburg (practical part at CMORE AUTOMOTIVE GMBH), 2019
Lectures
- Automatic Recognition of Arabic handwriting, part of "Angewandte Bildverarbeitung" (applied image processing), apl. Prof. Dr.-Ing. habil. Al-Hamadi
- Grundlagen der Signalorientierten Bildverarbeitung (in Vertretung)
Journals
-
Dinges, L.; Fiedler, M.-A.; Al-Hamadi, A.; Hempel, T.; Abdelrahman, A.; Weimann, J.; Bershadskyy, D. & Steiner, J. Exploring facial cues: automated deception detection using artificial intelligence Neural Computing and Applications, Springer, 2024, 1-27, DOI 10.1007/s00521-024-09811-x (ImpactFactor 6.0)
- Handrich, S.; Dinges, L.; Al-Hamadi, A; Werner, P; Saxen, F. and Al Aghbari, Z. Simultaneous prediction of valence / arousal and emotion categories and its application in an HRC scenario, Journal of Ambient Intelligence and Humanized Computing , vol. 12, no. 1, pp. 57–73, 2021.
- Dinges, L, Al-Hamadi, A.; Elzobi, M. & El-etriby, S. Synthesis of Common Arabic Handwritings to Aid Optical Character Recognition Research, Sensors , vol. 16, no. 3, p. 346, 2016.
- Dinges, L.; Al-Hamadi, A.; Elzobi, M.; El etriby, S. & Ghoneim, A. ASM based Synthesis of Handwritten Arabic Text Pages, Scientific World Journal, 2015
- Elzobi, M.; Al-Hamadi, A.; Al Aghbari, Z. & Laslo, D.IESK-ArDB: a database for handwritten Arabic and optimized topological segmentation approach International Journal on Document Analysis and Recognition, Springer, 2012
- Dinges, L.; Al-Hamadi, A.; Elzobi, M.; Al-Aghbari, Z. & Mustafa, H. Offline Automatic Segmentation based Recognition of Handwritten Arabic Words International Journal of Signal Processing, Image Processing and Pattern Recognition (IJSIP), 2011, 4, 131-144
Conference papers
-
Abdelrahman, A. A.; Hempel, T.; Khalifa, A.; Al-Hamadi, A. & Dinges, L. "L2cs-net: Fine-grained gaze estimation in unconstrained environments"
2023 8th International Conference on Frontiers of Signal Processing (ICFSP), 2023, 98-102 - Dinges, L.; Fiedler, M.-A.; Al-Hamadi, A.; Abdelrahman, A.; Weimann, J. & Bershadskyy, "Uncovering Lies: Deception Detection in a Rolling-Dice Experiment", Image Analysis and Processing -- ICIAP 2023, Springer Nature Switzerland, 2023, 293-303
- Hempel, T.; Dinges, L. & Al-Hamadi, A. Sentiment-based Engagement Strategies for intuitive Human-Robot Interaction arXiv preprint arXiv:2301.03867, VISIGRAPP (4: VISAPP), 2023
-
Ravindra, G.; D.; Dinges; L.; Al-Hamadi; A.; Baranau & V. "Fast and Precise Binary Instance Segmentation of 2D Objects for Automotive Applications" Computer Science Research Notes, Union Agency, Science Press, 3201, 302-305, 2022
- Hempel, T.; Fiedler, M.-A.; Khalifa, A.; Al-Hamadi, A. & Dinges, L. "Semantic-Aware Environment Perception for Mobile Human-Robot Interaction" 2021 12th International Symposium on Image and Signal Processing and Analysis (ISPA), 2021
- Dinges, L.; Al-Hamadi, A.; Hempel, T. & Al Aghbari, Z. Using Facial Action Recognition to Evaluate User Perception in Aggravated HRC Scenarios 2021 12th International Symposium on Image and Signal Processing and Analysis (ISPA), 2021, 195-199
- Handrich, S.; Dinges, L.; Al-Hamadi, A.; Werner, P. & Z. A. Aghbari, “Simultaneous Prediction of Valence/Arousal and Emotions on AffectNet, Aff-Wild and AFEW-VA,” in The 11th International Conference on Ambient Systems, Networks and Technologies (ANT 2020) / The 3rd International Conference on Emerging Data and Industry 4.0 (EDI40 2020) / Affiliated Workshops, April 6-9, 2020, Warsaw, Poland , 2020, vol. 170, pp. 634–641.
- Saxen, F.; Werner, P; Handrich, S; Othman, E.; Dinges, L. and Al-Hamadi, A; “Face Attribute Detection with MobileNetV2 and NasNet-Mobile,” 2019, pp. 176–180.
- Handrich, S.; Dinges, L.; Saxen, F. & Al-Hamadi, A, Simultaneous Prediction of Valence / Arousal and Emotion Categories in Real-time,” in IEEE International Conference on Signal and Image Processing Applications (ICSIPA), 2019.
- Dinges, L.; Al-Hamadi, A.; Elzobi, M. & and A. Nürnberger, Automatic Recognition of Common Arabic Handwritten Words based on OCR and N-grams, in International Conference on Image Processing, ICIP 2017, Beijing, China, September 17-20, pp. 3625–3629, 2017
- Dinges, L.; Al-Hamadi, A.; Elzobi, M. & El-etriby, S. Synthetic based Validation of Segmentation of Handwritten Arabic Words, Manuscript Cultures, 2015, 1, 10-18
- Dinges, L.; Al-Hamadi, A. & Elzobi, M. A Locale Group Based Line Segmentation Approach for Non Uniform Skewed and Curved Arabic Handwritings International Conference on Document Analysis and Recognition (ICDAR), 2013, 803-806
- Dinges, L.; Al-Hamadi, A. & Elzobi, M. An Approach for Arabic Handwriting Synthesis based on Active Shape Models International Conference on Document Analysis and Recognition (ICDAR), 2013, 1292-1296
- Dinges, L.; Al-Hamadi, A. & Elzobi, M. An Active Shape Model based approach for Arabic Handwritten Character Recognition The 11th International Conference on Signal Processing ( ICSP'2012), 2012, 1194-1198
- Dinges, L.; Elzobi, M.; Al-Hamadi, A. & Al-Aghbari, Z. Synthizing Handwritten Arabic Text Using Active Shape Models Image Processing and Communications Challenges 3, Springer, 2011, 102, 401-408
- Elzobi, M.; Al-Hamadi, A. & Dinges, L. A Hidden Markov Model-based Approach with an Adaptive Threshold Model for Off-line Arabic Handwriting Recognition International Conference on Document Analysis and Recognition (ICDAR), 2013, 945-949
- Elzobi, M.; Al-Hamadi, A.; Dinges, L & Michaelis., B. A structural feature based segmentation for off-line handwritten Arabic text 5th International Symposium on Image/Video Communication over Fixed and Mobile Networks, ISIVC 2010 . - IEEE, 2010, 1-4